Those Weird and Wonderful Benford Numbers
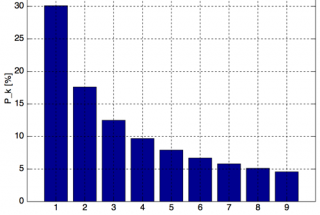
The Benford distribution of first digits
From the Report on Business Magazine, December 2010
When WikiLeaks released nearly 80,000 classified documents relating to military operations in Afghanistan this past July, a wide range of journalists, politicians and members of the public were eager to see the data. Some of them were looking for a true tally of IED attacks, others for the number of civilian casualties, and so on. One group, led by Drew Conway, a PhD candidate in political science at New York University, had a more unusual goal. They wanted to use an arcane statistical law to determine if the data in the reports was truly raw, or if anyone had tampered with it.
The fact that Conway could do so, and reach findings that are considered scientifically valid, has ramifications in many other fields, including forensic accounting and possibly finance.
The law is Benford’s Law, stated in 1938 by U.S. physicist Frank Benford. It posits that in lists of multi-digit numbers drawn from a wide range of natural and man-made phenomena, the leading digits aren’t distributed in a uniform way. You might expect the numbers 1 to 9 to appear with roughly equal frequency in the first slot. In fact, lower numbers are much more common in that position than higher numbers. The digit 1 appears first about a third of the time, 2 the next most often, and each subsequent number up to 9 appears with less frequency—9 is first only in about 1 in 20 cases. Data sets that adhere to the law are varied and surprising, and they include war casualties, lengths of rivers, areas of lakes, the size of craters on the Moon, even the byte sizes of files stored on your hard drive.
Benford’s Law is also pervasive in business. Stock prices and index levels, data from tax returns and expense claims all conform to the law if the numbers have not been fudged. That makes Benford’s Law a powerful tool in forensic data analysis. If someone cooks books, the falsified numbers will likely reveal themselves. That has lead to the emergence of experts like Dr. Mark Nigrini, a business professor at the College of New Jersey whose March 2011 book Forensic Analytics and Forensic Investigation will describe the methods and techniques of detecting fraud using statistical tools including Benford’s Law. Nigrini’s services are much in demand. He’s worked with the Brooklyn District Attorney’s Office, law firms, corporations and tax authorities on the application of Benford’s Law in fraud detection. That includes two occasions on which he’s advised Revenue Canada on what he describes as their “proactive” use of Benford’s in catching fraudulent income tax returns.
In a more recent case, Nigrini advised a “major international consumer goods manufacturer” on how to detect irregularities in coupon redemption by retailers. Coupon redemption is ripe for corruption, Nigrini tells me, because manufacturers can’t physically keep all the paper coupons involved. “There aren’t warehouses big enough to store them,” he says. So companies shred the coupons within weeks of receiving them and destroy what might later be crucial physical evidence. Faced with that reality, fraud detection must happen very quickly. And a system incorporating the Benford analysis of incoming coupon claims can provide crucial early warning of potential fraud.
Other experts have been trying to apply the law to scams more elaborate than bookkeeping or tax fraud. One of the great financial thrillers of our era was independent investigator Harry Markopolos’s cracking of the Madoff Investment Securities scam. In a now-famous memo Markopolos submitted to the SEC in 2005, “The World’s Largest Hedge Fund is a Fraud,” he argued that the consistently profitable investment results that Madoff had reported for almost two decades were impossible to achieve. Markopolos used so-called Mosaic Theory to arrive at his conclusions. Analyzed using Benford’s Law, interestingly, Madoff’s fraudulent numbers are so close to the predicted first digit distribution that some analysts, like Paul Kedrosky, have concluded that he cooked the books with Benford in mind.
What Kedrosky’s analysis points out is that, like all rules of thumb, Benford’s Law has its limits. You need a lot of numbers to be fudged for Benford analysis to catch the distribution error, for example, while many frauds are based on just one or two. More crucially, Benford will only work at the transaction level, not at the aggregated portfolio level. As one money manager explained to me, when many individual transaction prices are fake—as they were in Enron’s notorious “special purpose vehicles”– Benford can shine new light. But only Enron’s auditors had access to transaction data. Totals that appeared in its financial reports didn’t contravene Benford’s predicted distribution of first digits.
There’s also the more obvious problem of what happens when fraudsters get wise to Benford’s Law. Benford’s distribution of the frequency of first digits can now be found in Wikipedia and a variety of other sources. Anyone cooking books can create values that will conform to the law.
Nabbing a specific culpirt may be difficult as well. In the case of the Afghanistan data, Conway’s team didn’t fin strong evidence of tampering. But what if they had, I asked Conway. Would that have implied wrongdoing my military authorities or by Wikileaks in gathering and publishing the data?